A systematic review of data science and machine learning applications to the oil and gas industry
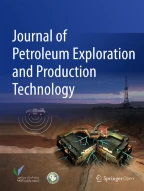
This study offered a detailed review of data sciences and machine learning (ML) roles in different petroleum engineering and geosciences segments such as petroleum exploration, reservoir characterization, oil well drilling, production, and well stimulation, emphasizing the newly emerging field of unconventional reservoirs. The future of data science and ML in the oil and gas industry, highlighting what is required from ML for better prediction, is also discussed. This study also provides a comprehensive comparison of different ML techniques used in the oil and gas industry. With the arrival of powerful computers, advanced ML algorithms, and extensive data generation from different industry tools, we see a bright future in developing solutions to the complex problems in the oil and gas industry that were previously beyond the grip of analytical solutions or numerical simulation. ML tools can incorporate every detail in the log data and every information connected to the target data. Despite their limitations, they are not constrained by limiting assumptions of analytical solutions or by particular data and/or power processing requirements of numerical simulators. This detailed and comprehensive study can serve as an exclusive reference for ML applications in the industry. Based on the review conducted, it was found that ML techniques offer a great potential in solving problems in almost all areas of the oil and gas industry involving prediction, classification, and clustering. With the generation of huge data in everyday oil and gas industry activates, machine learning and big data handling techniques are becoming a necessity toward a more efficient industry.
Similar content being viewed by others

Artificial intelligence techniques and their application in oil and gas industry
Article 16 November 2020
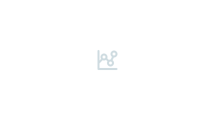
RETRACTED ARTICLE: A comprehensive study on artificial intelligence in oil and gas sector
Article 10 August 2021
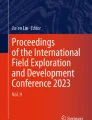
Knowledge-Based Machine Learning Approaches to Predict Oil Production Rate in the Oil Reservoir
Chapter © 2024
Explore related subjects
Avoid common mistakes on your manuscript.
Introduction
Artificial Intelligence (AI) is the field that integrates computational power with human intelligence to produce smart and reliable solutions to extremely nonlinear and highly complicated problems. AI is the field of science that allows computers to think and decide on their own. Machine learning (ML) is a subset of AI that provides statistical tools to explore and analyze big data. ML is comprised of further subsets such as supervised, unsupervised, and reinforced learning. Supervised learning is the data learning technique applied when some past or labeled data is available for future forecasting by function approximation. The unsupervised learning technique is the machine learning technique when the past labeled data is unavailable and is usually used for clustering purposes. Reinforced learning is the combination of supervised and unsupervised learning techniques in which some part of the data is labeled and some part is not.
In the last two decades, engineering journals have reported numerous articles utilizing ML for regression, function approximation, and classification problems. With the development of intelligent oilfields and big data technology, the adoption of the ML method has gained new vitality for the study of problems in the oilfield development process. With the advent of computing techniques, several correlations utilizing ML have come to the fore, especially in reservoir characterization (Anifowose 2012; Fatai A Anifowose et al. 2013a, b), reservoir engineering (Al-Marhoun and Osman 2002; Gharbi et al. 1999; Gharbi and Elsharkawy 1999); and reservoir geomechanics (Tariq et al. 2017a, b) and many other areas in petroleum engineering applications.
The most repeated question that ML petroleum researchers faced in their everyday life is that ML models are usually limited to the data set tested, so how to globalize this and produce more general correlations? ML applications have common limitations and challenges that hinder the globalization of the created models, such as overfitting, coincidence, excessive training, lack of interpretability of results, and bias. Besides, these models require a large amount of data that is not available in many cases.
Overfitting is considered the most common problem in ML applications. This is due to the lack of an appropriate amount of data to be used for training. To overcome this issue, the ratio of data points to the total number of weights used by the connections (ρ) was used to lessen the effect of insufficient data. The coincidence effect is another issue that accompanies the AI supervised learning models as they try to match a specific dataset, so there is a probability of getting a good match by coincidence. This also can happen in other regression analysis techniques, which require working on methods to minimize that occurrence (Livingstone et al. 1997). Overtraining can happen when there is no clear stopping stage for the training. The error may stay decreasing by updating the model structure, including the weights. The real risk, in that case, is that the model can be more complex to fit a specific dataset, becoming impossible to generalize after that. A training methodology named “early stopping” uses a control set that monitors the training process to overcome this. If error begins to rise, the early stopping will end the training process. Other techniques are being used to save time and effort, such as reinforcement learning with in-stream supervision, such as generative adversarial networks that monitor the learning of two competing networks to better understand the model concept (Hossain 2018).
The availability of large datasets is also a concern, which affects the training accuracy and goodness of the model. If the gathered data is limited, a methodology like single-shot learning is implemented, in which the AI model is pre-trained on a similar dataset and is enhanced with experience.
Interpretability is the key to data analysis. AI models are not that simple, and even in some cases, it is impossible to interpret the results even in modeling small linear problems. The single connections in the models do not alone affect results, but the whole combined connections do. One of the methods developed to help in that regard is the local interpretable model and its agnostic explanations, which try to detect which parts of the raw data the model depends on mostly for estimations. In the generalized additive models’ method, the separation between model features enhances each feature's interpretation.
The lack of AI models' generalization ability is a major limitation that delays the widespread of AI in the oil and gas industry. It is hard for many models to be used in circumstances different from those used in building the original model (Virginia 2018). Additional resources are to be utilized each time for training new datasets, even if they were similar to previous cases (Ramamoorthy and Yampolskiy 2018). The reusability of the ML models is also quite challenging. Usually, the trained models on one geological field are less reliable when applied to other geological fields. It is highly recommended to implement the model when the input parameters of the given dataset lay within the range of the input parameters on which the model is to be implemented (Mohaghegh 2017).
Lastly, the effect of bias cannot be ignored and sometimes is hard to be detected and mitigated. Many researchers are solving the issues related to AI bias by understanding the model's objective and its associated results. Using model-independent perturbations by substituting the inputs with random values obtained from a normal distribution will help avoid biases (Samek et al. 2018). Table 1 provides a summary of all limitations of AI and ML models.
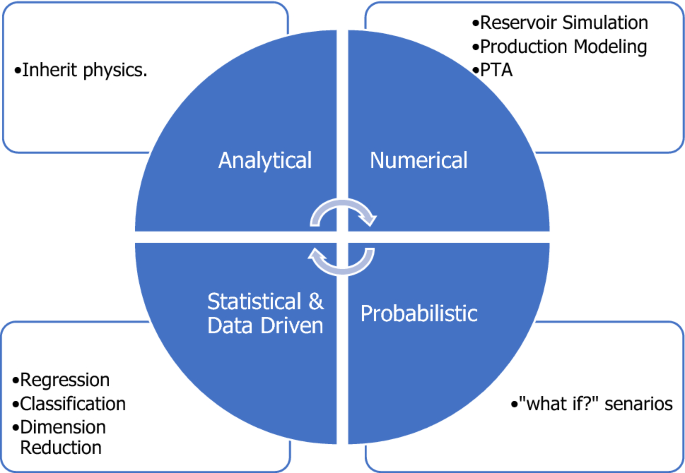
What is needed from AI in the oil and gas industry?
Many oil and gas industry giants are currently applying AI in oil and gas operations. AI advances made it suitable for several applications such as precision in drilling and automation, saving oil and gas producers' time and money. These advances are going to serve different aspects of the oil and gas industry, such as:
Precise drilling
Drilling activities are always accompanied by high risk and a high level of uncertainty. AI techniques coupled with the big data recorded by the smart sensors mounted on drilling strings such as pressure, temperature, and seismic surveys in real time can be used to overcome these challenges. Precise drilling using AI can enhance the control level of the rate of penetration and identify risks in advance.
Production optimization
Every oil and gas company focuses on production optimization and efficiency, which eventually increases profits with the help of AI, automated pattern recognition, and classification to prepare production data for generating analytics. Estimation and prediction models can then be built based on the refined data. It can also isolate the effects of the reservoir from the production control responses such as gas lift rates, choke openings, network routing, and artificial lift methods.
Reservoir management
Multiple teams from several aspects such as seismic, geology, reservoir, and production engineering are required to collaborate to achieve better reservoir management. The AI models can be trained with historical data of seismic surveys, geological descriptions, and production methodologies and then can be applied in the characterization or modeling of reservoirs and field monitoring.
Inspections
Frequent inspections are scheduled for detecting abnormal equipment performance to prevent failures of the equipment and potential accidents. That is why companies are looking for automated and smart detective approaches. Robots driven by AI models can help investigate abnormal equipment behavior by identifying anomalies using techniques such as pattern recognition. Besides, drones can inspect pipelines and offshore facilities that can detect, in real time, cracks or leaks in pipelines. They can also help in case of an emergency, such as gas leaks. In certain situations, these robots can intervene in emergency cases and use the procedure, which applies to that case, which will elevate the company's safety measures.
Chatbots
AI-powered chatbots can help engineers and scientists by digging in a database or archive of historical data, suggesting possible solutions to problems, providing correct standards of job execution, or help in teaching junior staff using natural language processing. Jacobs (2019) discussed three newly released chatbots in the oil and gas industry: Sandy, Nesh, and Ralphie. They are designed intentionally to provide answers to oil and gas professionals’ complex questions. These are also named virtual assistants that use artificial intelligence (AI) natural language processing (NLP), which has quickly entered the market through the tech giants Amazon, Apple, and Google, which enabled many millions of people to engage in dialogue with laptops, smartphones, and speakers.
Facilities monitoring
Intelligent cameras can reduce potential damage by detecting hazardous activities such as smoking in dangerous areas. They can be trained using photos and recordings of dangerous activities to alert the staff or take predefined actions. Moreover, they can detect if the employees are watering their protective PPE or not. Using this approach will help enhance safety management.
Commonly used machine learning techniques in oil and gas industry
Several ML techniques such as ANN, FL, SVM, DT, RF, KNN, RNN, CNN, and fuzzy C-means clusters are widely used in different applications of oil and gas. Table 4 summarizes some of the algorithms with their advantages and disadvantages.
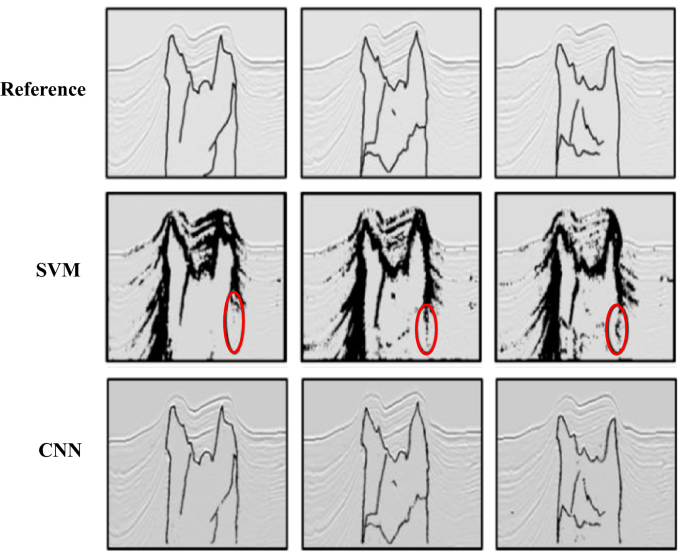
Petrofacies classification and fractures identification
Reservoir rocks can be classified and grouped based on their reservoir quality. Such classification can be done based on petrophysical rock properties (e.g., porosity, permeability, and pore size) and geological features (e.g., textures, diagenetic overprints, and pore types). Petrofacies are usually defined based on combining both petrophysical and geological attributes, which can be an essential tool for reservoir characterization (Avseth and Mukerji 2002). Petrofacies classification is frequently done using both core samples and wireline log data. Cores are not frequently available from all wells due to the time and cost associated, and thus several studies (Bhattacharya and Mishra 2018; Qi and Carr 2006; Sebtosheikh and Salehi 2015) have examined how machine learning algorithms can be trained on data obtained from certain cored well and then used to perform petrofacies classification in other un-cored wells. Petrofacies labels, defined as a function of depth based on the integration of well-log and core data, are used to train the models (Sebtosheikh and Salehi 2015; Silva et al. 2015). The utilized logs for facies identification are usually Gama Ray (GR), resistivity (Rt), neutron (NPHI), density (RHOB), and lithology (PEF). In addition, other features could be extracted from these logs to improve the prediction, such as total organic matter (TOC), matrix grain density (RHOMAA), and apparent volumetric cross-section (UMA).
Earlier studies have used ANN, SVM, and RF to classify petrofacies from well logs in both sandstone and carbonate reservoirs (Silva et al. 2015; Al-Anazi and Gates 2010; Martinelli et al. 2013; Salehi and Honarvar 2014). Nevertheless, more recent studies have suggested that Gradient Boosting (GB) algorithm outperforms ANN and SVM, especially when a limited number of features are available (Silva et al. 2015). Another algorithm that has shown success is the Random Forest (RF), which reduces the computational time for the training phase compared to GB (Bhattacharya and Mishra 2018). Based on the existing literature, it seems that there is no consensus regarding the most suitable machine learning technique for petrofacies classification. This could be due to several factors, including the wide variations in the features selected or available data, as well as differences in terms of complex geology and reservoir heterogeneity. Indeed, as pointed out by Silva et al. (Silva et al. 2015), the applicability of various algorithms has to be tested for each training/testing data set to be used. One major challenge that remains for the success of machine learning in this application is to have/select the right petrophysical and geological attributes/features to distinguish between facies. Such tasks remain mainly subjective and far from being automated or objective.
Fractures and facies identification are usually made through personal judgments based on field log and laboratory core analysis data. Recently, AI has been used to identify fractures and facies in unconventional formations. Tian and Daigle (2019) could identify micro-fractures and organic matter in siliceous and carbonate-rich shale samples and find the association between them using AI. That was to automate the process of understanding micro-fractures in shale samples to make it fast and avoid personal evaluations. SEM and EDS images were used to find fractures and organic matters in intact and deformed samples. The single-shot detector (SSD) deep learning approach was used to train the data obtained from the images. Around 97% of fractures in intact samples and 92% in deformed ones were identified using SSD. Also, detected organic matter images were overlapped over detected fractures to find the associations. It was found the clear majority of micro-fractures penetrated the OM and clay minerals. It seems that the combination of the soft OM and clay and brittle materials (quartz and calcite) enhances the fracability according to the study.
Well correlation
Correlating different reservoir units and formation tops across different wells is essential in reservoir characterization and modeling. Such a task may require significant time from experienced geologists, especially in large fields with hundreds of wells. The use of machine learning to handle this issue has been recognized many years back (Luthi and Bryant 1997). An interpreter has first to pick formation tops and perform well correlations in several wells, which will be used as a training dataset to perform interpretation in tens to hundreds of other wells. An increasing body of studies (Maniar et al. 2018; Zheng et al. 2019) has demonstrated that a deep convolutional neural network (CNN) can provide an accurate and efficient approach for well-log correlations. The most common log data used for the correlation includes gamma ray and resistivity, although any other geophysical well-log data with sufficient log character can be used. One crucial observation documented by Zheng et al. (2019) was the drastic reduction in prediction accuracy as the number and percentage of the training dataset decreases. This might be explained by the complexity of geology that would require wells covering different depositional environments and stratigraphic sequences throughout a field.
To produce a “universal” model for well correlation, Brazell et al. (2019) developed a deep CNN architecture trained based on five million data points derived from thousands of well-log and experienced interpreter correlations. The data was obtained from various depositional environments and basins within the USA. The authors have implemented a 3D search logic to determine the marker propagation pathway and the optimum correlation. The model does require some interpreted-top examples to be provided from the specific dataset to account for particular complexity within the geology of a given area. Nevertheless, no need for extensive training data set from the specific field due to the rich dataset used to build the model. The model could provide an accuracy of around 96% on the testing dataset. It is important to note that more interpreted examples might be needed for the training if the model is to be applied to a dataset outside the US with very different regional complex geology. Another potential consideration is incorporating seismic sequence stratigraphy into the workflow, which currently relies only on well-log data. This can be important, especially in benching out strata and faulted reservoirs where the spatial continuation of a given unit might be heterogonous.
Reservoir characterization
Machine learning has an increasing number of applications in the field of geosciences. Still, we focus here on applications directly related to reservoir characterization in the oil/gas industry. The areas discussed are petrophysical properties prediction from the seismic, core, and well-log data. Other properties such as water saturation, petroleum geochemical parameters, and reservoir geomechanics will be predicted.
Petrophysical properties prediction
Reservoir characterization plays a critical role in the oil and gas industry, such as developing optimal production and reservoir management strategies. Permeability, which determines the ability and direction of oil flow, is central in reservoir characterization. An accurate permeability determination is essential for material balance calculations, reservoir flow simulation, estimating oil production rate, stimulation strategies, and enhancing oil recovery. However, permeability is very difficult to determine due to its complexity and highly nonlinear nature. Therefore, machine learning techniques are widely used to predict petrophysical parameters such as porosity, permeability, capillary pressure, relative permeability, and bulk density. Table 5 shows a summary of the studies used to predict porosity and permeability.
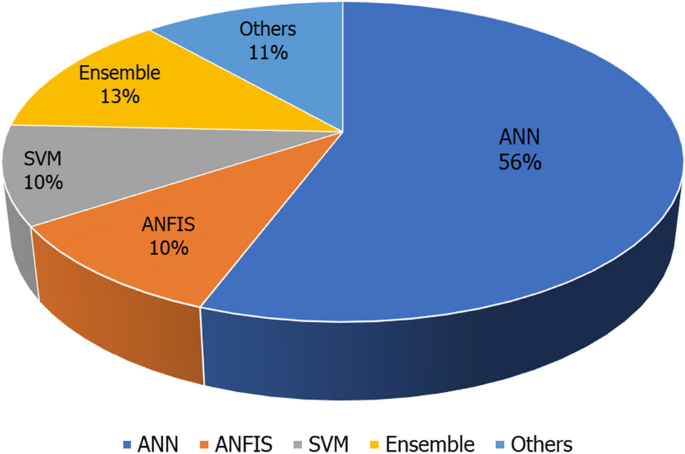
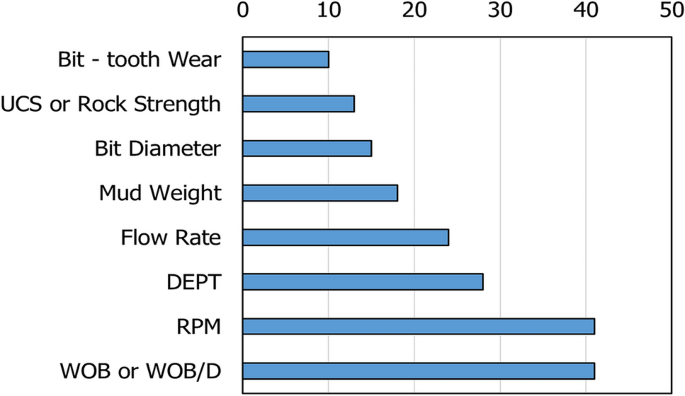
Ahmed et al. (2019) presented a comparative study of predicting ROP using several intelligence techniques. ROP was predicted for two wells using an extreme learning machine, ANN, and SVR techniques. They selected the input parameters for the ROP models based on the specific energy concept. The ROP was predicted for more than 8800 data points based on the RPM, WOB, torque, depth, mud weight, flow rate, nozzle sizes, and standpipe pressure (SPP). They reported that all ROP models showed acceptable prediction performance with a correlation coefficient higher than 0.70 for the testing data. However, among all tested techniques, support vector regression showed the best ROP estimation with a correlation coefficient of 0.94.
Mehrad et al. (2020) used a machine learning approach to develop a rigorous ROP model for vertical wells. They used different parameters to determine the ROP, including logging, drilling, and geomechanical parameters. They found that the best ROP prediction can be obtained by using the uniaxial compressive strength (UCS), mudflow rate, weight on bit (WOB), Depth, mud density (MD), and revolutions per minute (RPM) as input parameters. After that, they combined the least-squares support vector machines (LSSVM) with different optimization algorithms to estimate the ROP profile. The examined optimization algorithms are genetic algorithms (GA), particle swarm optimization (PSO), and cuckoo optimization algorithm (COA). LSSVM-GA, LSSVM-PSO, and LSSVM-COA hybrid algorithms were used to predict the ROP for two vertical wells, and more than 2000 data points were used to train and tests the hybrid models. LSSVM-COA showed the best prediction performance for training and testing wells among all tested algorithms, and an R-square of around 0.802 was achieved.
Artificial intelligence showed an effective approach for estimating the drilling performance, and accurate profiles of ROP can be predicted. However, it is noticeable that there is a lack of implementation of those techniques for real-time operations, especially for gas wells. Also, most of the available ANN-based models were developed to predict the ROP for a certain section, usually for the reservoir section. No attempt was reported for predicting the full profile of ROP using the ANN technique. Predicting the complete profile of ROP in real time can significantly improve the drilling performance and reduce the operational time and cost.
Furthermore, the coupling of different drilling efficiency indicators can help in improving the drilling operations by considering more than one parameter. For example, the ROP models can be coupled with the MSE concept to determine the best drilling conditions in drilling time (ROP) and required drilling energy (MSE). Hassan et al. (2018) coupled the torque modeling with the mechanical specific energy (MSE) to optimize the drilling performance. First, artificial intelligent techniques were used to predict the torque and ROP profiles for around 18000 ft. Then, the MSE was calculated for the whole drilling section using the surface drilling parameters. After that, the MSE was coupled with the torque and ROP profiles to identify the optimum drilling conditions that will result in maximizing the ROP and minimizing the required drilling energy (MSE). They mentioned that the developed approach would enable the drilling engineers to evaluate and optimize the drilling performance in real-time applications; hence, the surface drilling parameters can be controlled to maintain the drilling operations within the optimum conditions.
Besides, AI techniques were used to estimate several drilling problems, such as loss of circulation, one of the most common drilling problems that can increase the overall drilling cost by around 25–40%. Solomon et al. (2017) developed a new ANN model to estimate the loss circulation zones. The developed model can also recommend the suitable sizes of loss circulation materials based on the characteristics of the depleted zones. They used 30 case studies to train and validate the developed ANN model. They mentioned that the ANN model showed a very acceptable prediction performance, and a coefficient of determination of 0.8 was obtained. Besides, they compared the reliability of the developed model with different fracture predictive models, and they concluded that the developed ANN model could reduce the estimation error from around 26% to less than 16%.
Manshad et al. (2017) used an SVM and radial basis function to assess the loss of circulation problems for 30 oil wells. They reported that SVM showed high performance in predicting the amount of loss circulation material required to overcome the thief zones. A coefficient of determination of 0.8 was obtained between the predicted results and actual field data. In comparison, the radial basis function was able to estimate the mitigation of loss of circulation problems with an accuracy of 78.3%.
Al-Hameedi et al. (2018) estimated the volume of lost circulation materials for 500 wells using the machine learning technique. They predicted the volume of fluid losses based on the profiles of mud weight, bit nozzle sizes, ROP, equivalent circulation density (ECD), plastic viscosity (PV), and WOB. They reported that the machine learning models were able to predict the volume of fluid losses with very acceptable error for different types of mud loss, including partial, seepage, severe, and total mud losses.
Alkinani et al. (2020) used an ANN technique to predict the volume of drilling fluids losses during drilling fractured zones. They developed and validated the ANN model using 1500 wells. Also, the lost circulation volume was determined based on the profiles of mudflow rate, yield point (YP), PV, ECD, bit nozzle sizes, RPM, and WOB. They reported that the ANN model was able to predict the loss of circulation with a coefficient of determination higher than 0.92.
Abbas et al. (2019) applied SVM and ANN techniques to estimate the severity of loss of circulation while drilling. They used 1120 case studies from 385 wells to train and validate the new AI models for different types of mud losses such as seepage, partial, severe, and total fluids losses. They used the rock lithology, mud properties, and drilling surface parameters to predict the severity of loss of circulation. They reported that the developed ANN model was able to estimate the fluids loss with a correlation coefficient higher than 0.82. While the SVM model showed better prediction performance compared to the ANN model, a correlation coefficient higher than 0.91 was obtained.
Overall, different AI techniques were utilized to estimate the loss of circulation problems. ANN and SVM methods are the common AI tools that are used for this purpose. The very practical performance was reported for predicting the loss circulation based on the mud properties, rock lithology, and drilling parameters. However, the application of these models in real-time operation might be restricted due to the huge drilling data, leading to misleading results or delaying the model prediction. Therefore, proper data cleaning could be required to improve the data quality and reduce the data size for problems in real-time applications (Elkatatny et al. 2016).
Drilling fluids
Drilling is one of the most critical tasks, with challenges including lost circulation, clogged pipes, wellbore instability, and kicks occurring regularly. Drilling fluid, sometimes known as the "blood of the drill," is a direct or indirect remedy to the challenges stated above during the drilling process. It helps to keep the wellbore clean and retain the wellbore's integrity. For instance, high mud weight controls the high wellbore pressures and prevents kicks. On the other hand, high mud weight has a tendency to frack the formation. Similarly, low mud weight prevents fractures but can cause kick or blowout. Further drilling fluids prevent the pipe from sticking during drilling by building thin filter cake on the wellbore wall as well as by removing drilling cuttings out the wellbore. The drilling fluid works as an architect for the wellbore. The operation's success or failure is largely determined by the drilling fluid's performance and compatibility (Agwu et al. 2018). Many drilling issues can be avoided by using the proper drilling fluids. Drilling fluids are always chosen based on data analysis and expertise gained from previously drilled wells in the area. Each well design includes a drilling fluid program that specifies drilling fluid, additives, rheology, density, filtration, and other drilling fluid parameters. Combating wellbore difficulties involves comprehensive analysis and decision-making to build the drilling fluid to satisfy specific needs that suit distinct formation features.
The majority of drilling fluid design is done in the laboratory through trial and error. Hence, a system that can use existing data and provide a deeper knowledge of drilling fluid is required. Machine learning models are created using the parameters of drilling fluids and the downhole circumstances. These models aid in forecasting changes in drilling fluid parameters and recommend the optimum course of action. Rheological models express a mathematical relationship between the shear rate and the shear stress to describe the fluid flow behavior. This relationship is complicated in the case of drilling fluids. However, no single rheological model can accurately fit all drilling fluids' shear stress-shear rate data across all shear rate ranges. Instead, a plethora of mathematical models with varying degrees of relevance has been utilized. These mathematical models do not precisely capture the behavior of non-Newtonian fluids. For instance, the Bingham plastic model does not describe the drilling fluid flow behavior at a low shear rate. Further, it overestimates the yield point of the drilling fluid. The power-law model does not account for the yield point of drilling fluids. There are challenges in performing hydraulic calculations due to many rheological parameters involved in the case of the Herschel-Bulkley model (Huang et al. 2020).
Regression approaches are utilized to predict rheological proficiencies such as an ANN. For greater accuracy, the ANN model can be trained continuously with more data sets. It gives a more comprehensive view of how to comprehend the drilling performance. For example, if there is a reduction in pump pressure during the drilling operation, which happens for several reasons, including thinning effect on the drilling fluid, quick transport of the cuttings to the surface, reservoir fluid influx in the wellbore, and lost circulation, etc. Here AI interlinks different parameters, improves the decision-making process, and brings back the engineers on the right track within a short time.
Tables 8 and 9 outlines several studies of artificial intelligence in drilling fluids. The tables summarize the drilling fluids properties investigated and the AI technique used. They also show the input and output parameters and accuracy of a performance evaluation using correlation coefficient (R2), mean square error (MSE), average absolute percent relative error (AAPE), etc.
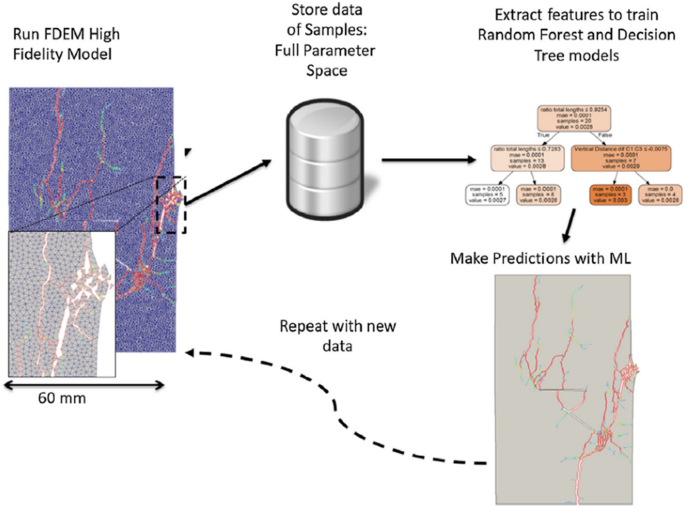
Fluid flow through a fracture network is challenging to simulate because of the structural complexity. Srinivasan et al. (2018) built a machine learning tool to predict the solute flow through a fractured network. A discrete fracture network (DFN) methodology was used to simulate fluid flow in fracture networks. Solute flow through the fracture network is usually taking the shortest path. Graph theory was used to reduce the number of fractures to those that only contribute to flow. Then, SVM and RF were used to identify the backbone of the fracture network that contributes to flow. This significantly reduced the computational power when simulating flow using the DNF model. The trained model could capture the early solute breakthrough precisely; nevertheless, it was not as useful in predicting late time flow.
Proppant distribution in a hydraulic fracture is crucial information as it could be used to optimize MSF design. Maity et al. (2019) identified proppant particles from cored samples based on imaging processes supported by machine learning classification tools. The goal was to understand the proppant distribution after an MSF job. This helps identify the location of the new infill wells to be drilled and the completion spacing as proppant distribution can tell the length of popped fracture and which clusters were propped. Images were taken for the particles obtained from a 600 ft cored interval using a dedicated slanted well to obtain these cores. Training ANN classification, the particles were divided into proppant, calcite, and others. The following attributes of particles were used as input: hue, roundness, size, darkness, roughness, translucence, and entropy. K-fold cross-validation was used for hidden layer size optimization for ANN. It was benchmarked against other classifiers such as SVM. It was concluded that the proppant is limited within 30 ft vertical distance in the studied formation. It was validated against field data using other classification techniques.
AI is also an active area in hydraulic fracture design optimization such as the number of horizontal wells, number of stages, volume of proppant and fluids, type of chemical additives, and sweet spot identification (Awoleke and Lane 2011; Lolon et al. 2016). Most of the AI developed models ignore important geological and reservoir properties such as porosity, permeability, saturation, and pressure. These data are challenging to obtain especially along the horizontal sections of the wellbore. Some researchers replaced these data with the location of the well (i.e., coordinates) as the mentioned properties are spatially changing (Mishra et al. 2015; Wang and Chen 2019). Wang and Chen (2019) trained machine learning algorithms (RF, SVM, ANN, and AdaBoost) on 3160 horizontal well data of Montney unconventional formation to predict the first-year production and optimize the fracture design. Features such as proppant mass, well location, lateral length, fluids treatment size and type, completion type, number of stages were used for training. Recursive feature elimination with cross-validation (RFECV) was used to find the most significant features where RF was used for prediction. Then, algorithms were trained based on the most important features to predict the production rate from a fractured well. Using RFECV showed that the most important parameter in enhancing production is the mass of proppant pumped for the case of Montney formation and the location of the well. It was found that using more than the four features (proppant mass, latitude, longitude, and TVD) will not improve the correlation coefficient. It was also observed that the RF results in the best performance in terms of prediction accuracy. One drawback of the trained model is its lack of reservoir properties such as permeability, porosity, and pressure.
Optimization of hydraulic fracture stages using gradient-free (i.e., AI) methods has been applied by many researchers (Iino et al. 2020; Yu and Sepehrnoori 2013). The objective function that is usually optimized is the net present value (NPV) or cumulative production. Features such as fracture half-length, spacing, porosity, permeability, the distance between laterals, and fracture conductivity were used for the optimization. Different AI algorithms were tried such as covariance matrix adaptation evolution strategy (CMA-ES), simultaneous perturbation stochastic approximation (SPSA), genetic algorithm (GA), and non-dominated sorting genetic algorithm (NSGA-II). Rahmanifard and Plaksina (2018) aimed to optimize hydraulic fracture stages in unconventional gas formation based on cumulative production or NPV using AI-based optimization tools such as GA, Differential Evolution (DE), and Particle Swarm Optimization (PSO). Gradient-based methods are usually used for optimization purposes. However, they suffer from being trapped in local optima which means that the absolute optima could not be found. Also, many functions could not be differentiated at a certain value or range. Hence, this study was utilizing AI-based optimization tools that are gradient-free. The authors used Wattenbarger et al. (1998) analytical slap model to estimate gas cumulative flow within a certain production period. The optimization function is the NPV which is a function of the cumulative gas production, water cumulative production, and cost of hydraulic fracturing and waste disposal. The objective is to find the optimum number of hydraulic fractures (NHFs) that will maximize NPV. The PSO outperformed the other AI methods such as DE and GA as it required much fewer iteration for convergence.
Du et al. (2017) utilized embedded discrete fracture modeling (EDFM) to train an AI-based algorithm to estimate productivity in the Permian Basin. Authors used EDFM for fracture representation in a reservoir simulator; a method that reduces the need for using fine grids. The EDFM composes of two elements: matrix and fracture that can be represented separately. Mangrove which is a commercial software was used for hydraulic fracture network generation. AI was implemented to remove unnecessary fracture complexity that would not contribute to productivity. Using AI methods to reduce the complexity of the fracture and then implement it in EDFM resulted in significant simulation time reduction as compared only to using Mangrove. It enabled doing sensitivity analysis as it was feasible. However, the simplified structure resulted because the AI should be history matched to tune parameters such as reservoir permeability otherwise an error up 40% could be the outcome.
Bhattacharya et al. (2019) used machine learning algorithms to predict production in fractured Marcellus shale. The authors used the data of one well with 28 stages of hydraulic fractures in Marcellus shale to predict the production rate. The data used were petrophysical and geomechanical data (GR, sonic), pressure data (surface, casing, tubing), and fiber optics data such as distributed acoustic sensing (DAS) and distributed temperature sensing (DTS) while missing are hydraulic fracturing data and design. Ghahfarokhi et al. (2018) also implemented DAS and two years of DTS data for estimating production from Marcellus shale well. Bhattacharya et al. (2019) implemented the following machine learning tools: RF, ANN, and SVM. Feature engineering was implemented to find secondary attributes from the row data such as the brittleness index (BI). Collinearity analysis was implemented to find the most suitable features which reduced them from 34 originally to 18. All models could predict the production rate to good accuracy. However, SVM provided less accuracy with more computation time. Including hydraulic fracturing, reservoir, and PVT properties should improve accuracy. The model's lack of these data is a major limitation of their approach. Figure 6 shows that the Poisson ratio (PR) and brittleness index (BI) were the most important while DAS and DTS were not as significant.
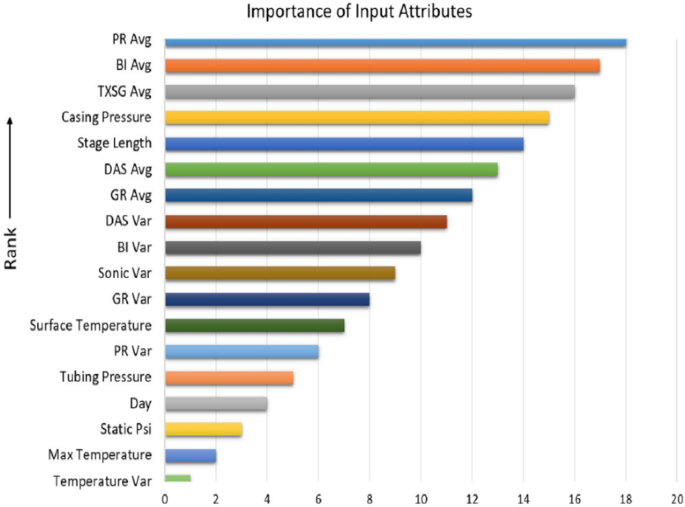
Similar concepts were applied to other shale formations such as Bakken shale. Luo et al. (2019) investigated the possibility of predicting the productivity of horizontally drilled wells in Bakken shale based on completion and geological parameters. Geology and completion data of 2061 horizontal wells in the Bakken were used. These include vertical depth, amount of proppant, water saturation, porosity, permeability thickness…etc. Spearman correlation, RF, and joint mutual information (JMI) were used for feature selection. Deep learning (ANN) was used as a predictive model based on one-year production data. Based on feature selection, it was found that the formation thickness, depth, and amount of proppant are the most important parameters to predict the production in the first year. It was also observed that less porous spots require more proppant to increase productivity which agrees with the physics of unconventional. Wang et al. (2011) applied AI on 2780 MSF and 139 vertical wells in the Bakken to predict productivity. A deep neural network was used in the study with k-fold cross-validation to check the predictiveness of the model. The number of hidden layers and neurons was optimized to give the best prediction for 6 and 18 months. The model showed that the amount of proppant placed in each stage is the most important parameter in predicting productivity. The trained model resulted in a small root mean square error (RMSE) when predicting the 6 and 18 months of production.
Sweet spots identification in unconventional formation is an important process as horizontal drilling combined with MSF is an expensive process that should be justified by good productivity. Also, unconventional formations cover large areas, and hence, finding the right location to complete the well is critical. Tahmasebi et al. (2017) defined the sweet spots as the ones having high TOC and fracability index (FI). They used multiple linear regression (MLR) to train log data from shale formation to predict the TOC and FI. Nonlinear models such as FL, hybrid neural networks (NN)/FL, GA were also implemented. For variable selection, stepwise selection was implemented. Mineralogy composition was used to assess the fracability where quartz is the brittle mineral. MLR failed to predict FI where the correlation coefficient was 0.44 which is an unsatisfactory value. The prediction of TOC was better where the correlation coefficient was around 0.88. The hybrid (NN + FL) machine learning, among nonlinear models, (HML) could provide better accuracy and remove the weakly correlated variables.
Rastogi and Sharma (2019) used machine learning tools to find the impact of fracturing chemicals on production using one-year production data. Different algorithms were used for feature selection such as F-Regression, Decision tree-based regressions, recursive feature elimination … etc. The data were obtained from different fracture jobs in the Powder River Basin. Chemicals additives were found to be in the top 5 parameters that impact productivity out of 11 selected features.
AI has also been applied to the area of acid fracturing in terms of conductivity prediction. Acid self-prop the fracture by generating peaks and valleys that act as a conduit for the fluids to follow. Akbari et al. (2017) used a 106 data point generated experimentally to develop a conductivity correlation based on GA. The developed correlation resulted in better accuracy as compared to the popular correlations for acid fracture conductivity. Eleibide et al. (2018) applied ANN and adaptive network-based fuzzy inference systems on the same data set. The authors showed that the model accuracy was improved as compared to Akbari et al.'s model. Desouky et al. (2020a, 2020b) utilized more than 500 data points to generate a more accurate acid fracture conductivity correlation that considers rock type and etching pattern.
Future and challenges
The utilization of ML techniques to handle a large data set and to predict several parameters in many aspects of the oil and gas industry is rapidly growing. The main reason behind that is the generation of large data in everyday activates of the oil and gas industry. To be able to process the large data and make it useful, a careful data processing and handling has to take place and ML techniques are a great tool to do that. Furthermore, due to the complexity of the different relationships between the many factors controlling the productivity of an oil or gas well, ML techniques are widely used to figure out these complex relationships and build a multilayered correlation to relate the different factors. Without ML, the classical liner/nonlinear regression methods do not have the capability to handle high complexity as ML models do. Also, the high uncertainty of the many oil and gas industry activates is a major concern given the capital-intensive nature of these activities, building a reliable forecast and prediction models are necessary to navigate through these challenges while optimizing the outcomes.
ML techniques have provided many solutions to the oil and gas industry to thrive. At the same time, there are many disadvantages of these models that are sometimes ignored or rarely mentioned. One of the main disadvantages of using ML in building a relationship between several parameters is whenever there is a high correlation, it does not necessary imply causation (“correlation does not imply causation”). Building a high correlation model linking several parameters together based on the data used should not be taken as indication that these parameters are truly having a cause/effect relationship unless there is a proven physical or scientific relationship between them. Many developed models in the literature fail to address this fact and tend to associate correlation with causation. Another common challenge facing the applicability of ML techniques is the availability and accuracy of data used to build and test these models. The data has to be accurate in order to produce a useful model. Otherwise, the model developed will never be useful no matter its high accuracy. Conducting data collection quality assurance is highly recommended to avoid this issue.
A common criticism of ML models is that they require a large and diverse data set to train the model. Any model needs a sufficient representative data in order to capture the underlying structure that allows it to generalize to new similar cases. For instance, a ML model built to predict production of a certain formation would only be applicable for that formation and under the same conditions when the training data it collected. Generalizing the predictive models has to be done with careful consideration of the constrain of these models and the diversity and inclusivity of the data used to build them. This is a major disadvantage of ML techniques as they tend to be generalized without careful consideration of this limitation.
Surly, ML cannot be used to predict anything related to oil and gas industry or build a correlation between any two or more parameters. Before undergoing building the relationships between the different factors, a scientific and factual explanation of the actual “physical” relationship between these parameters has to be addressed first. Also, using ML to predict and forecast based on historical data has to be done carefully by addressing and assuring that the future conditions are similar to the historical events. ML tend to be a very useful tool to deal with big data and to build the complex relationships between the different parameters that linear/nonlinear regression models cannot handle. Many of the correlation that has been established based on regression analysis of laboratory data are being replaced with correlation developed using ML methods that are more case specific rather than general correlations.
Deep learning which is a subset of ML based on ANN is very efficient for many tasks but it is not the solution to every problem as it faces many challenges. Deep learning algorithms need to be trained with large sets of data and the access and availability of accurate data is not always possible in many aspects of the oil and gas industry. Therefore, overfitting is considered the most common problem in ML applications which is mainly due to the lack of an appropriate amount of data to be used for training. Also, overtraining can happen when there is no clear stopping stage for the training and the error keeps decreasing by updating the model structure and the model become more complex to fit a specific dataset. Even when dealing with large data sets, a major challenge is the training cost. In many situations, supercomputers are needed to handle large oil and gas data sets to build and run ML models.
The future trend of ML applications in the oil and gas industry looks promising. With the arrival of the internet of things and the automation of many of the oil and gas activates and the high reliance on data, it is possible to minimize risks and enhance productivity by integrating ML algorithms that are continuously trained and enhanced using the continuous flow of data. With the generation of large data in oil and gas industry, petroleum engineers and geoscientists must be exposed to big data handling techniques that are being developed in the AI domain. Making the most of the availability of data is something being addressed nowadays and will continue to be the trend for the future. Optimization cannot be reached without the utilization of the powerful capabilities of AI.
Concluding remarks
Based on the review of the literature and the authors’ work on the applications of AI in petroleum engineering, the following remarks can be made:
- AI offers a huge potential in solving problems in almost all areas of the oil and gas industry involving prediction, classification, and clustering. Compared to other areas of engineering, petroleum engineering and geosciences have special relevance because of two important factors. Firstly, we deal with nature rather than man-made materials and processes. Variation in rocks, oil, and brine cannot be easily handled by any closed-form solution. The employment of numerical methods in such a situation is too cumbersome as well as unrealistic. Secondly, the amount of data produced every day from cores, logs, and seismic exploration in conventional reservoirs to multistage hydraulic fracturing in unconventional reservoirs is too huge to be interpreted properly using classical approaches.
- The biggest challenge for researchers is to have access to laboratory and/or field data. Oil companies should come forward to share data in whatever secured form acceptable to benefit the literature from the enormous potential that AI is ready to offer.
- With the arrival of the internet of things and the live relay of data from drilling and production facilities, it is possible to minimize risks and enhance production by integrating AI algorithms trained based on past data.
- There is a degree of uncertainty in the data coming out from the laboratories, logs, or seismic data. The depth record of the samples on which the measurements are taken may not be exact due to depth shifts, and the log data may not exactly represent the property corresponding to that depth point as it involves the averaging of the properties of layers that are intersected within the logging sensors such as transmitters and receivers. Seismic data also averages vast volumes of rocks in a given block. As a result, a significant amount of data collected during the drilling operation is unreliable. Consequently, data cleaning and uncertainty quantification is another huge area that needs to be integrated with AI to develop realistic solutions to the problems.
- The solution to a problem using AI is rarely guaranteed if one attempts to relate the input with the target data without a thorough understanding of the physics of the problem. The major challenge in this approach is setting up the problem so that the algorithm can easily connect input and the target data. Wherever possible, information from analytical models should be suitably used in the input data to help the AI model arrive at the solution efficiently and more quickly.
- Problems involving the prediction of curves such as in viscosity-pressure curve in PVT data require extensive exploration of algebraic equations in addition to the understanding of the physics of the problem.
- Challenges remain in AI tools, too, in overfitting, coincidence effect, overtraining, etc. It is hoped that researchers in soft computing will develop modified and/or new AI tools.
- With the generation of huge data in petroleum engineering from cores to logs to seismic exploration, the petroleum engineers and geoscientists must be exposed to normal as well as big data handling techniques that are being developed in the AI domain. Exposure to AI tools should start right from their undergraduate education.
References
- Aadnoy BS, Fazaelizadeh M, Hareland G (2010) A 3D analytical model for wellbore friction. J Can Pet Technol. https://doi.org/10.2118/141515-PAArticleGoogle Scholar
- Abbas AK, Al-haideri NA, Bashikh AA (2019) Implementing artificial neural networks and support vector machines to predict lost circulation. Egypt J Pet 28(4):339–347. https://doi.org/10.1016/j.ejpe.2019.06.006ArticleGoogle Scholar
- Abdelgawad K, Elkatatny S, Mousa T, Mahmoud M, Patil S (2018) Real time determination of rheological properties of spud drilling fluids using a hybrid artificial intelligence technique. J Energy Resour Technol. https://doi.org/10.2118/192257-MSArticleGoogle Scholar
- Abdi Y, Garavand AT, Sahamieh RZ (2018) Prediction of strength parameters of sedimentary rocks using artificial neural networks and regression analysis. Arab J Geosci 11:587. https://doi.org/10.1007/s12517-018-3929-0ArticleGoogle Scholar
- Abdulraheem A, Ahmed M, Vantala A, Parvez T, (2009) Prediction of rock mechanical parameters for hydrocarbon reservoirs using different artificial intelligence techniques. In: Society of petroleum engineers—SPE Saudi Arabia section technical symposium 2009. Society of Petroleum Engineers. https://doi.org/10.2118/126094-ms
- Adesina FAS, Abiodun A, Anthony A, Olugbenga F (2015) Modeling the effect of temperature on environmentally safe oil based drilling mud using artificial neural network algorithm. Pet Coal 57(1):60–70 Google Scholar
- Agwu OE, Akpabio JU, Alabi SB, Dosunmu A (2018) Artificial intelligence techniques and their applications in drilling fluid engineering: a review. J Pet Sci Eng 167:300–315. https://doi.org/10.1016/j.petrol.2018.04.019ArticleGoogle Scholar
- Ahmadi MA, Chen Z (2019) Comparison of machine learning methods for estimating permeability and porosity of oil reservoirs via petro-physical logs. Petroleum 5:271–284. https://doi.org/10.1016/j.petlm.2018.06.002ArticleGoogle Scholar
- Ahmadi MA, Pournik M, Shadizadeh SR (2015) Toward connectionist model for predicting bubble point pressure of crude oils: application of artificial intelligence. Petroleum 1:307–317. https://doi.org/10.1016/j.petlm.2015.08.003ArticleGoogle Scholar
- Ahmadi MA, Shadizadeh SR, Shah K, Bahadori A (2018) An accurate model to predict drilling fluid density at wellbore conditions. Egypt J Pet 27:1–10. https://doi.org/10.1016/j.ejpe.2016.12.002ArticleGoogle Scholar
- Ahmed A, Ali A, Elkatatny S, Abdulraheem A (2019) New artificial neural networks model for predicting rate of penetration in deep shale formation. Sustain. https://doi.org/10.3390/su11226527ArticleGoogle Scholar
- Akbari M, Ameri MJ, Kharazmi S, Motamedi Y, Pournik M (2017) New correlations to predict fracture conductivity based on the rock strength. J Petr Sci Eng 152:416–426. https://doi.org/10.1016/j.petrol.2017.03.003ArticleGoogle Scholar
- Al-Anazi A, Gates ID (2010) On the capability of support vector machines to classify lithology from well logs. Nat Resour Res. https://doi.org/10.1007/s11053-010-9118-9ArticleGoogle Scholar
- Al-Bulushi NI, King PR, Blunt MJ, Kraaijveld M (2012) Artificial neural networks workflow and its application in the petroleum industry. Neural Comput Appl 21:409–421. https://doi.org/10.1007/s00521-010-0501-6ArticleGoogle Scholar
- Al-Hajri NM, Al-Ghamdi A, Tariq Z, Mahmoud M (2020) Scale-prediction/inhibition design using machine-learning techniques and probabilistic approach. SPE Prod Oper 35:0987–1009. https://doi.org/10.2118/198646-PAArticleGoogle Scholar
- Al-Hameedi AT, Alkinani HH, Dunn-Norman S, Flori RE, Hilgedick SA, Amer AS, Alsaba MT (2018) using machine learning to predict lost circulation in the rumaila field, Iraq. In: Paper presented at the SPE Asia pacific oil and gas conference and exhibition, Brisbane, Australia. https://doi.org/10.2118/191933-MS
- Al-Marhoun MA, Osman EA (2002) Using artificial neural networks to develop New PVT correlations for saudi crude oils. In: Society of petroleum engineers—Abu Dhabi international petroleum exhibition and conference 2002, ADIPEC 2002. Society of Petroleum Engineers, Abu Dhabi. https://doi.org/10.2523/78592-ms
- Al-Shammasi AA (2001) A review of bubblepoint pressure and oil formation volume factor correlations. SPE Res Eval Eng 4:146–160. https://doi.org/10.2118/71302-PAArticleGoogle Scholar
- Alarifi SA, Miskimins J (2021) A new approach to estimating ultimate recovery for multistage hydraulically fractured horizontal wells by utilizing completion parameters using machine learning. SPE Prod Oper 36:468–483. https://doi.org/10.2118/204470-PAArticleGoogle Scholar
- Alarifi S, AlNuaim S, Abdulraheem A (2015) Productivity index prediction for oil horizontal wells using different artificial intelligence techniques. In: All days. SPE. https://doi.org/10.2118/172729-MS
- Alkinani HH, Al-Hameedi ATT, Dunn-Norman S, Lian D (2020) Application of artificial neural networks in the drilling processes: can equivalent circulation density be estimated prior to drilling? Egypt J Pet. https://doi.org/10.1016/j.ejpe.2019.12.003ArticleGoogle Scholar
- Ameen MS, Smart BGD, Somerville JM, Hammilton S, Naji NA (2009) Predicting rock mechanical properties of carbonates from wireline logs (A case study: Arab-D reservoir, Ghawar field, Saudi Arabia). Mar Pet Geol 26:430–444. https://doi.org/10.1016/j.marpetgeo.2009.01.017ArticleGoogle Scholar
- Amiri M, Zahedi G, Yunan MH (2015) Water saturation estimation in tight shaly gas sandstones by application of progressive quasi-static (PQS) algorithm—a case study. J Nat Gas Sci Eng 22:468–477. https://doi.org/10.1016/j.jngse.2014.12.027ArticleGoogle Scholar
- Amiri M, Salamati A, Hatampour A, Rostami A, Heidari M (2014) Application of progressive quasistatic (PQS) algorithm in prediction of water saturation in tight gas sandstones—A case study. In: 20th Formation evaluation symposium of Japan 2014
- Andrea TA, Kalayeh H (1991) Applications of neural networks in quantitative structure-activity relationships of dihydrofolate reductase inhibitors. J Med Chemi 34(9):2824–2836. https://doi.org/10.1021/jm00113a022ArticleGoogle Scholar
- Anemangely M, Ramezanzadeh A, Amiri H, Hoseinpour S-A (2019) Machine learning technique for the prediction of shear wave velocity using petrophysical logs. J Pet Sci Eng 174:306–327. https://doi.org/10.1016/j.petrol.2018.11.032ArticleGoogle Scholar
- Anifowose F, Abdulraheem A (2011) Fuzzy logic-driven and SVM-driven hybrid computational intelligence models applied to oil and gas reservoir characterization. J Nat Gas Sci Eng. https://doi.org/10.1016/j.jngse.2011.05.002ArticleGoogle Scholar
- Anifowose FA, Abdulraheem A, Al-Shuhail A, Schmitt DP (2013a) Improved permeability prediction from seismic and log data using artificial intelligence techniques. SPE Middle East Oil Gas Show Conf MEOS Proc 3:2190–2196. https://doi.org/10.2118/164465-msArticleGoogle Scholar
- Anifowose FA, Labadin J, Abdulraheem A (2013b) Prediction of petroleum reservoir properties using different versions of adaptive neuro-fuzzy inference system hybrid models. Int J Comput Inf Syst Ind Manag Appl 5:413–426 Google Scholar
- Anifowose FA, Labadin J, Abdulraheem A (2017) Ensemble machine learning: an untapped modeling paradigm for petroleum reservoir characterization. J Pet Sci Eng 151:480–487. https://doi.org/10.1016/j.petrol.2017.01.024ArticleGoogle Scholar
- Anifowose FA (2012) Advances in hybrid computational intelligence application in oil and gas reservoir characterization. In: Society of petroleum engineers—SPE Saudi Arabia section young professionals technical symposium 2012, YPTS 2012. pp. 1–8. https://doi.org/10.2118/160921-ms
- Arabjamaloei R, Shadizadeh S (2011) Modeling and optimizing rate of penetration using intelligent systems in an Iranian southern oil field (ahwaz oil field). Pet Sci Technol. https://doi.org/10.1080/10916460902882818ArticleGoogle Scholar
- Ariturk MS (2019) Optimizing the production and injection wells flow rates in geothermal field using artificial intelligence. In: Environmental science
- Aulia A, Rahman A, Quijano Velasco JJ (2014) Strategic well test planning using random forest. In: All days. SPE. https://doi.org/10.2118/167827-MS
- Avseth P, Mukerji T (2002) Seismic lithofacies classification from well logs using statistical rock physics. Petrophysics 43:70–81 Google Scholar
- Awoleke OO, Lane RH (2011) Analysis of data from the barnett shale using conventional statistical and virtual intelligence techniques. SPE Reserv Eval Eng. https://doi.org/10.2118/127919-PAArticleGoogle Scholar
- Ba alawi M, Gharbi S, Mahmoud M (2020) Modeling and prediction of resistivity, capillary pressure and relative permeability using artificial neural Network. In: Day 3 Wed, January 15, 2020. IPTC. https://doi.org/10.2523/IPTC-19854-MS
- Baarimah SO, Abdulraheem A, Anifowose FA (2014) Artificial intelligence (AI) techniques for predicting the reservoir fluid properties of crude-oil systems. In: Society of petroleum engineers—international petroleum technology conference 2014, IPTC 2014—innovation and collaboration: keys to affordable energy. pp. 3953–3968
- Bageri BS, Anifowose FA, Abdulraheem A (2015) Artificial intelligence based estimation of water saturation using electrical measurements data in a carbonate reservoir. In: SPE middle east oil and gas show and conference, MEOS, Proceedings. Society of Petroleum Engineers, pp. 499–515. https://doi.org/10.2118/172564-ms
- Bagheri A, Nazari A, Sanjayan J (2019) The use of machine learning in boron-based geopolymers: function approximation of compressive strength by ANN and GP. Meas J Int Meas Confed. https://doi.org/10.1016/j.measurement.2019.03.001ArticleGoogle Scholar
- Bahorich M, Farmer S (1995) The coherence cube. Lead Edge 14(10):1053–1058 ArticleGoogle Scholar
- Bahorich MS, Farmer SL (1995) 3-D seismic discontinuity for faults and stratigraphic features: the coherence cube. In: 1995 SEG annual meeting. pp. 93–96. https://doi.org/10.1190/1.1887523
- Barbosa LFFM, Nascimento A, Mathias MH, de Carvalho JA (2019) Machine learning methods applied to drilling rate of penetration prediction and optimization: a review. J Pet Sci Eng 183:106332. https://doi.org/10.1016/j.petrol.2019.106332ArticleGoogle Scholar
- Bas CL (2016) Frugal innovation, sustainable innovation, reverse innovation; why do they look alike? Why are they different? J Innov Econ Manag 21:9–26 ArticleGoogle Scholar
- Berthelot A, Solberg AHS, Gelius LJ (2013) Texture attributes for detection of salt. J Appl Geophys. https://doi.org/10.1016/j.jappgeo.2012.09.006ArticleGoogle Scholar
- Bhattacharya S, Ghahfarokhi PK, Carr TR, Pantaleone S (2019) Application of predictive data analytics to model daily hydrocarbon production using petrophysical, geomechanical, fiber-optic, completions, and surface data: a case study from the Marcellus Shale, North America. J Petrol Sci Eng 176:702–715 ArticleGoogle Scholar
- Bhattacharya S, Mishra S (2018) Applications of machine learning for facies and fracture prediction using bayesian network theory and random forest: case studies from the Appalachian basin. USA J Pet Sci Eng 170:1005–1017. https://doi.org/10.1016/j.petrol.2018.06.075ArticleGoogle Scholar
- Bilgesu HI, Tetrick LT, Altmis U, Mohaghegh S, Ameri S (1997) New approach for the prediction of rate of penetration (ROP) values. In: Proceedings—SPE eastern regional conference and exhibition. https://doi.org/10.2523/39231-ms
- da Bispo VDS, Scheid CM, Calcada LA, da Meleiro LAC (2017) Development of an ANN-based soft-sensor to estimate the apparent viscosity of water-based drilling fluids. J Pet Sci Eng. https://doi.org/10.1016/j.petrol.2016.11.030ArticleGoogle Scholar
- Bondi G, Creamer R, Ferrari A, Fenton O, Wall D (2018) Using machine learning to predict soil bulk density on the basis of visual parameters: tools for in-field and post-field evaluation. Geoderma 318:137–147. https://doi.org/10.1016/j.geoderma.2017.11.035ArticleGoogle Scholar
- Boschert S, Rosen R (2016). Digital twin-the simulation aspect. In: Mechatronic futures: challenges and solutions for mechatronic systems and their designers. https://doi.org/10.1007/978-3-319-32156-1_5
- Bourgoyne AT, Young FS (1999) A multiple regression approach to optimal drilling and abnormal pressure detection. SPE Repr Ser. https://doi.org/10.2118/4238-paArticleGoogle Scholar
- Brazell S, Bayeh A, Ashby M, Burton D (2019) A Machine-learningbased approach to assistive well-log correlation. Petrophysics 60:469–479. https://doi.org/10.30632/PJV60N4-2019a1ArticleGoogle Scholar
- Buhulaigah A, Al-Mashhad AS, Al-Arifi SA, Al-Kadem MS, Al-Dabbous MS (2017) Multilateral wells evaluation utilizing artificial intelligence. In: SPE middle east oil & gas show and conference. Society of petroleum engineers. https://doi.org/10.2118/183688-MS
- Chen F, Duan Y, Zhang J, Wang K, Wang W (2015) Application of neural network and fuzzy mathematic theory in evaluating the adaptability of inflow control device in horizontal well. J Pet Sci Eng 134:131–142 ArticleGoogle Scholar
- Cheshmeh Sefidi A, Ajorkaran F (2019) A novel MLP-ANN approach to predict solution gas-oil ratio. Pet Sci Technol 37:2302–2308. https://doi.org/10.1080/10916466.2018.1490759ArticleGoogle Scholar
- Chhantyal K, Viumdal H, Mylvaganam S (2017) Ultrasonic level scanning for monitoring mass flow of complex fluids in open channels—a novel sensor fusion approach using AI techniques. In: Proceedings of IEEE sensors. https://doi.org/10.1109/ICSENS.2017.8234010
- Van Dao D, Ly HB, Trinh SH, Le TT, Pham BT (2019) Artificial intelligence approaches for prediction of compressive strength of geopolymer concrete. Materials (basel). https://doi.org/10.3390/ma12060983ArticleGoogle Scholar
- Deosarkar MP, Sathe VS (2012) Predicting effective viscosity of magnetite ore slurries by using artificial neural network. Powder Technol. https://doi.org/10.1016/j.powtec.2011.12.058ArticleGoogle Scholar
- Desouky M, Tariq Z, Aljawad MS, Alhoori H, Mahmoud M, AlShehri D (2020a) Data-driven acid fracture conductivity correlations honoring different mineralogy and etching patterns. ACS Omega 5(27):16919–16931. https://doi.org/10.1021/acsomega.0c02123ArticleGoogle Scholar
- Desouky M, Aljawad MS, Alhoori H, Al-Shehri D (2020b) Evaluating the effects of acid fracture etching patterns on conductivity estimation using machine learning techniques. In: Paper presented at the SPE Europec, Virtual. https://doi.org/10.2118/200527-MS
- Devi PRS, Baskaran R, Abirami S (2015) Multi-label learning with class-based features using extended centroid-based classification technique (CCBF). Procedia Comput Sci 54:405–411. https://doi.org/10.1016/j.procs.2015.06.047ArticleGoogle Scholar
- Di H, Gao D (2014) Gray-level transformation and Canny edge detection for 3D seismic discontinuity enhancement. Comput Geosci 72:192–200. https://doi.org/10.1016/j.cageo.2014.07.011ArticleGoogle Scholar
- Di H, Gao D (2016) Improved estimates of seismic curvature and flexure based on 3D surface rotation in the presence of structure dip. Geophysics. https://doi.org/10.1190/GEO2015-0258.1ArticleGoogle Scholar
- Di H, Wang Z, AlRegib G (2018) search and discovery.com; Deep convolutional neural networks for seismic salt-body delineation. (AAPG annual convention & exhibition)
- Du S, Liang B, Yuanbo L (2017) Field study: embedded discrete fracture modeling with artificial intelligence in permian basin for shale formation, In: SPE annual technical conference and exhibition. Society of petroleum engineers. https://doi.org/10.2118/187202-MS
- Dupriest FE, Koederitz WL (2005) Maximizing drill rates with real-time surveillance of mechanical specific energy. In: All days. SPE. https://doi.org/10.2118/92194-MS
- Eleibide M, Hassan AM, Mahmoud M, Abdulraheem A, Elkatatny S (2018) Intelligent prediction of acid-fracturing performance in carbonates reservoirs. Society of Petroleum Engineers—SPE Kingdom of Saudi Arabia Annual Technical Symposium and Exhibition 2018, SATS 2018,
- Elkatatny S, Mahmoud M, Tariq Z, Abdulraheem A (2018a) New insights into the prediction of heterogeneous carbonate reservoir permeability from well logs using artificial intelligence network. Neural Comput Appl 30:2673–2683. https://doi.org/10.1007/s00521-017-2850-xArticleGoogle Scholar
- Elkatatny S, Tariq Z, Mahmoud M (2016) Real time prediction of drilling fluid rheological properties using artificial neural networks visible mathematical model (white box). J Pet Sci Eng 146:1202–1210. https://doi.org/10.1016/j.petrol.2016.08.021ArticleGoogle Scholar
- Elkatatny S, Tariq Z, Mahmoud M, Abdulraheem A (2018b) New insights into porosity determination using artificial intelligence techniques for carbonate reservoirs. Petroleum 4:408–418. https://doi.org/10.1016/j.petlm.2018.04.002ArticleGoogle Scholar
- Elkatatny S, Tariq Z, Mahmoud M, Abdulraheem A, Mohamed I (2019) An integrated approach for estimating static Young’s modulus using artificial intelligence tools. Neural Comput Appl 31:4123–4135. https://doi.org/10.1007/s00521-018-3344-1ArticleGoogle Scholar
- El-Sebakhy EA (2009) Forecasting PVT properties of crude oil systems based on support vector machines modeling scheme. J Petrol Sci Eng 64(1–4):25–34. https://doi.org/10.1016/j.petrol.2008.12.006ArticleGoogle Scholar
- Evangelatosorn GI, Michael L. Payne, BP plc (2016) “Advanced BHA-ROP modeling including neural network analysis of drilling performance data”, IADC/SPE178852-MS, Fort Worth, Texas
- Feifei C, Yonggang D, Junbin ZW, Weifeng W (2015) Application of neural network and fuzzy mathematic theory in evaluating the adaptability of inflow control device in horizontal well. J Pet Sci Eng. https://doi.org/10.1016/j.petrol.2015.07.020ArticleGoogle Scholar
- Fertl WH, Hammack GW (1971) Comparative look at water saturation computations in shaly pay sands. Soc. Petrophysicists Well-Log Anal
- Ganji-Azad E, Rafiee-Taghanaki S, Rezaei H, Arabloo M (2014) Hossein Ali Zamani, Reservoir fluid PVT properties modeling using adaptive neuro-fuzzy inference systems. J Nat Gas Sci Eng 21:951–961. https://doi.org/10.1016/j.jngse.2014.10.009ArticleGoogle Scholar
- Gharbi RBC, Elsharkawy AM (1999) Neural network model for estimating the PVT properties of middle east crude oils. SPE Reserv Eval Eng 2:255–265. https://doi.org/10.2118/56850-PAArticleGoogle Scholar
- Gharbi RB, Elsharkawy AM, Karkoub M (1999) Universal neural-network-based model for estimating the PVT properties of crude oil systems. Energy Fuels 13:454–458. https://doi.org/10.1021/ef980143vArticleGoogle Scholar
- Gholanlo HH, Amirpour M, Ahmadi S (2016) Estimation of water saturation by using radial based function artificial neural network in carbonate reservoir: a case study in Sarvak formation. Petroleum 2:166–170. https://doi.org/10.1016/j.petlm.2016.04.002ArticleGoogle Scholar
- Gidh Y, Purwanto A, Bits S (2012) Artificial neural network drilling parameter optimization system improves ROP by predicting/managing bit wear. Paper presented at the SPE Intelligent Energy International, Utrecht, The Netherlands. https://doi.org/10.2118/149801-MS
- Gowida A, Elkatatny S, Al-Afnan S, Abdulraheem A (2020) New computational artificial intelligence models for generating synthetic formation bulk density logs while drilling. Sustainability 12:686. https://doi.org/10.3390/su12020686ArticleGoogle Scholar
- Guitton A, Wang H, Trainor-Guitton W (2017) Statistical imaging of faults in 3D seismic volumes using a machine learning approach. https://doi.org/10.1190/segam2017-17589633.1
- Hale D (2013) Methods to compute fault images, extract fault surfaces, and estimate fault throws from 3D seismic images. Geophysics. https://doi.org/10.1190/GEO2012-0331.1ArticleGoogle Scholar
- Hassan A, Abdulraheem A, Elkatatny S, Ahmed M (2017) New approach to quantify productivity of fishbone multilateral well. In: Proceedings—SPE annual technical conference and exhibition. https://doi.org/10.2118/187458-ms
- Hassan A, Al-Majed A, Elkatatny S, Mahmoud M, Abdulraheem A, Nader M, Abughaban M, Khamis M (2018) Developing an efficient drilling system by coupling torque modelling with mechanical specific energy. In: Paper presented at the SPE Kingdom of Saudi Arabia Annual Technical Symposium and Exhibition, Dammam, Saudi Arabia. https://doi.org/10.2118/192251-MS
- Hassan A, Mahmoud M, Al-Majed A, Abdulraheem A (2020) A new technique to quantify the productivity of complex wells using artificial intelligence tools. In: Paper presented at the international petroleum technology conference, Dhahran, Kingdom of Saudi Arabia. https://doi.org/10.2523/IPTC-19706-Abstract
- He J, Tang M, Hu C, Tanaka S, Wang K, Wen X, Yusuf N (2021) Deep reinforcement learning for generalizable field development optimization. SPE J. https://doi.org/10.2118/203951-PAArticleGoogle Scholar
- Hegde C, Gray K (2018) Evaluation of coupled machine learning models for drilling optimization. J Nat Gas Sci Eng. https://doi.org/10.1016/j.jngse.2018.06.006ArticleGoogle Scholar
- Helle HB, Bhatt A (2002) Fluid saturation from well logs using committee neural networks. Pet Geosci 8:109–118. https://doi.org/10.1144/petgeo.8.2.109ArticleGoogle Scholar
- Hemmati-Sarapardeh A, Shokrollahi A, Tatar A, Gharagheizi F, Mohammadi AH, Naseri A (2014) Reservoir oil viscosity determination using a rigorous approach. Fuel 116:39–48. https://doi.org/10.1016/j.fuel.2013.07.072ArticleGoogle Scholar
- Hoang M (2016) Tuning of viscosity and density of non-Newtonian fluids through mixing process using multimodal sensors, sensor fusion and models. 156
- Hossain M (2018) Frugal innovation: a review and research agenda. J Clean Prod 182:926–936 ArticleGoogle Scholar
- Hu Y, Ding Y, Wen F, Liu L (2019) Reliability assessment in distributed multi-state series-parallel systems. Energy Procedia 159:104–110. https://doi.org/10.1016/j.egypro.2018.12.026ArticleGoogle Scholar
- Hua Y, Guo J, Zhao H (2015) Deep belief networks and deep learning. In: Proceedings of 2015 international conference on intelligent computing and internet of things. IEEE, pp. 1–4. https://doi.org/10.1109/ICAIOT.2015.7111524
- Huang L, Dong X, Clee TE (2017) A scalable deep learning platform for identifying geologic features from seismic attributes. Lead Edge. https://doi.org/10.1190/tle36030249.1ArticleGoogle Scholar
- Huang Y, Zheng W, Zhang D, Xi Y (2020) A modified Herschel-Bulkley model for rheological properties with temperature response characteristics of poly-sulfonated drilling fluid. Energy Sour Part A Recover Util Environ Eff 42:1464–1475. https://doi.org/10.1080/15567036.2019.1604861ArticleGoogle Scholar
- Iino A, Jung HY, Onishi T, Datta-Gupta A (2020) Rapid simulation accounting for well interference in unconventional reservoirs using fast marching method. https://doi.org/10.15530/urtec-2020-2468
- Jacobs T (2019) The oil and gas chat bots are coming. J Pet Technol 71:34–36. https://doi.org/10.2118/0219-0034-JPTArticleGoogle Scholar
- Jafari Kenari SA, Mashohor S (2013) Robust committee machine for water saturation prediction. J Pet Sci Eng 104:1–10. https://doi.org/10.1016/j.petrol.2013.03.009ArticleGoogle Scholar
- Jeirani Z, Mohebbi A (2006) Artificial neural networks approach for estimating filtration properties of drilling fluids. J Japan Pet Inst 49:65–70. https://doi.org/10.1627/jpi.49.65ArticleGoogle Scholar
- Jui-Sheng C, Chien-Kuo C, Mahmoud F, Ismail A-T (2011) Optimizing the prediction accuracy of concrete compressive strength based on a comparison of data-mining techniques. J Comput Civ Eng 25:242–253. https://doi.org/10.1061/(ASCE)CP.1943-5487.0000088ArticleGoogle Scholar
- Kamalyar K, Sheikhi Y, Jamialahmadi M (2012) Using an artificial neural network for predicting water saturation in an iranian oil reservoir. Pet Sci Technol 30:35–45. https://doi.org/10.1080/10916461003752561ArticleGoogle Scholar
- Kar S, Das S, Ghosh PK (2014) Applications of neuro fuzzy systems: a brief review and future outline. Appl Soft Comput 15:243–259. https://doi.org/10.1016/j.asoc.2013.10.014ArticleGoogle Scholar
- Kewalramani MA, Gupta R (2006) Concrete compressive strength prediction using ultrasonic pulse velocity through artificial neural networks. Autom Constr. https://doi.org/10.1016/j.autcon.2005.07.003ArticleGoogle Scholar
- Khamidy NI, Tariq Z, Syihab Z (2019) Development of ANN-based predictive model for miscible CO2 flooding in sandstone reservoir. In: SPE middle east oil and gas show and conference, MEOS, Proceedings. Society of petroleum engineers. https://doi.org/10.2118/194726-MS
- Khashman A, Akpinar P (2017) Non-destructive prediction of concrete compressive strength using neural networks. Procedia Comput Sci. https://doi.org/10.1016/j.procs.2017.05.039ArticleGoogle Scholar
- Khazaeni Y, Mohaghegh SD (2011) Intelligent production modeling using full field pattern recognition. SPE Reserv Eval Engi 14(6):735–749. https://doi.org/10.2118/132643-PAArticleGoogle Scholar
- Kumar A, Ridha S, Ganet T, Vasant P, Ilyas SU (2020) Machine learning methods for herschel-bulkley fluids in annulus: pressure drop predictions and algorithm performance evaluation. Appl Sci. https://doi.org/10.3390/app10072588ArticleGoogle Scholar
- Lecampion B, Bunger A, Zhang X (2018) Numerical methods for hydraulic fracture propagation: a review of recent trends. J Nat Gas Sci Eng. https://doi.org/10.1016/j.jngse.2017.10.012ArticleGoogle Scholar
- Liu H, Lang B (2019) Machine learning and deep learning methods for intrusion detection systems: a survey. Appl Sci 9:4396. https://doi.org/10.3390/app9204396ArticleGoogle Scholar
- Livingstone DJ, Manallack DT (1993) Statistics using neural networks: chance effects. J Med Chem 36(9):1295–1297. https://doi.org/10.1021/jm00061a023ArticleGoogle Scholar
- Livingstone D, Manallack D, Tetko I (1997) Data modelling with neural networks: advantages and limitations. J Comput Aided Mol Des 11:135–142. https://doi.org/10.1023/A:1008074223811ArticleGoogle Scholar
- Lolon E, Hamidieh K, Weijers L, Mayerhofer M, Melcher H, Oduba O (2016) Evaluating the relationship between well parameters and production using multivariate statistical models: a middle bakken and three forks case history. In: Day 3 Thu, February 11, 2016. SPE. https://doi.org/10.2118/179171-MS
- Luo G, Tian Y, Bychina M, Ehlig-Economides C (2019) Production-strategy insights using machine learning: application for bakken shale. SPE Reservoir Eval Eng 22(3):800–816 ArticleGoogle Scholar
- Luthi SM, Bryant ID (1997) Well-log correlation using a back-propagation neural network. Math Geol 29:413–425. https://doi.org/10.1007/BF02769643ArticleGoogle Scholar
- Maghrabi S, Kulkarni D, Teke K, Kulkarni SD, Jamison D (2014) Modeling of shale-erosion behavior in aqueous drilling fluids. In: Society of petroleum engineers—European unconventional resources conference and exhibition 2014: unlocking European potential. https://doi.org/10.2118/167691-ms
- Maity D, Ciezobka J (2019) Designing a robust proppant detection and classification workflow using machine learning for subsurface fractured rock samples post hydraulic fracturing operations. J Petrol Sci Eng 172:588–606. https://doi.org/10.1016/j.petrol.2018.09.062ArticleGoogle Scholar
- Maniar H, Ryali S, Kulkarni MS, Abubakar A (2018) Machine-learning methods in geoscience. In: SEG technical program expanded abstracts 2018. Society of exploration geophysicists, pp. 4638–4642. https://doi.org/10.1190/segam2018-2997218.1
- Manshad A, Rostami H, Niknafs H, Mohammadi A (2017) Integrated lost circulation prediction in oil field drilling operation. Research Gate 978–1–53610–852–1
- Mardi M, Nurozi H, Edalatkhah S (2012) A water saturation prediction using artificial neural networks and an investigation on cementation factors and saturation exponent variations in an Iranian oil well. Pet Sci Technol 30:425–434. https://doi.org/10.1080/10916460903452033ArticleGoogle Scholar
- Marfurt KJ, Kirlin RL, Farmer SL, Bahorich MS (1998) 3-D seismic attributes using a semblance-based coherency algorithm. Geophysics DOI 10(1190/1):1444415 Google Scholar
- Martinelli G, Eidsvik J, Sinding-Larsen R, Rekstad S, Mukerji T (2013) Building bayesian networks from basin-modelling scenarios for improved geological decision making. Pet Geosci. https://doi.org/10.1144/petgeo2012-057ArticleGoogle Scholar
- Masoudi R, Mohaghegh SD, Yingling D, Ansari A, Amat H, Mohamad N, Sabzabadi A, Dipak M (2020) Subsurface analytics case study; reservoir simulation and modeling of highly complex offshore field in malaysia, using artificial intelligent and machine learning. In: SPE annual technical conference and exhibition, October 2020. https://doi.org/10.2118/201693-MS
- Matin SS, Farahzadi L, Makaremi S, Chelgani SC, Sattari G (2018) Variable selection and prediction of uniaxial compressive strength and modulus of elasticity by random forest. Appl Soft Comput 70:980–987. https://doi.org/10.1016/j.asoc.2017.06.030ArticleGoogle Scholar
- Mehrad M, Bajolvand M, Ramezanzadeh A (2020) Jalil Ghavidel Neycharan, developing a new rigorous drilling rate prediction model using a machine learning technique. J Petrol Sci Eng 192:107338. https://doi.org/10.1016/j.petrol.2020.107338ArticleGoogle Scholar
- Melville PD, Guruswamy S (2002) Integration of 3D seismic with a reservoir model for reservoir characterisation. In: Abu Dhabi international petroleum exhibition and conference. https://doi.org/10.2118/78511-MS
- Miri R, Sampaio JHB, Afshar M, Lourenco A (2007) Development of artificial neural networks to predict differential pipe sticking in iranian offshore oil fields. In: International oil conference and exhibition in Mexico. Society of petroleum engineers. https://doi.org/10.2118/108500-MS
- Mishra S, Schuetter J, Zhong M, Lafollette R (2015) Data analytics for production optimization in unconventional reservoirs. https://doi.org/10.15530/urtec-2015-2167005
- Mnati KH, Hadi HA (2018) Prediction of penetration rate and cost with artificial neural network for alhafaya oil field. Iraqi J Chem Pet Eng 19:21–27. https://doi.org/10.31699/IJCPE.2018.4.3ArticleGoogle Scholar
- Mohaghegh S (2000) Virtual-intelligence applications in petroleum engineering: part 3—fuzzy logic. J Pet Technol 52:82–87. https://doi.org/10.2118/62415-JPTArticleGoogle Scholar
- Mohaghegh S (2011) Reservoir simulation and modeling based on artificial intelligence and data mining (AI & DM). J Nat Gas Sci Eng 3(6):697–705. https://doi.org/10.1016/j.jngse.2011.08.003ArticleGoogle Scholar
- Mohaghegh SD (2017) Shale analytics: data-driven analytics in unconventional resources. Springer International Publishing, Cham. https://doi.org/10.1007/978-3-319-48753-3BookGoogle Scholar
- Mollajan A, Memarian H, Jalali MR (2013) Prediction of reservoir water saturation using support vector regression in an Iranian carbonate reservoir. In: 47th US rock mechanics/geomechanics symposium 2013. pp. 1872–1877
- Moore BA, Rougier E, O’Malley D, Srinivasan G, Hunter A, Viswanathan H (2018) Predictive modeling of dynamic fracture growth in brittle materials with machine learning. Comput Mater Sci. https://doi.org/10.1016/j.commatsci.2018.01.056ArticleGoogle Scholar
- Murray AS, Cunningham RA (1955) Effect of mud column pressure on drilling rates. Trans AIME. https://doi.org/10.2118/505-gArticleGoogle Scholar
- Murtaza M, Mahmoud M, Tariq Z (2020) Experimental investigation of a novel, efficient, and sustainable hybrid silicate system in oil and gas well cementing. Energy Fuels 34:7388–7396. https://doi.org/10.1021/acs.energyfuels.0c01001ArticleGoogle Scholar
- Naderpour H, Rafiean AH, Fakharian P (2018) Compressive strength prediction of environmentally friendly concrete using artificial neural networks. J Build Eng. https://doi.org/10.1016/j.jobe.2018.01.007ArticleGoogle Scholar
- Najibi AR, Ghafoori M, Lashkaripour GR, Asef MR (2015) Empirical relations between strength and static and dynamic elastic properties of Asmari and Sarvak limestones, two main oil reservoirs in Iran. J Pet Sci Eng 126:78–82. https://doi.org/10.1016/j.petrol.2014.12.010ArticleGoogle Scholar
- Navrátil J, King A, Rios J, Kollias G, Torrado R, Codas A (2019) Accelerating physics-based simulations using end-to-end neural network proxies: an application in oil reservoir modeling. Front Big Data 2:33. https://doi.org/10.3389/fdata.2019.00033ArticleGoogle Scholar
- Negara A, Ali S, AlDhamen A, Kesserwan H, Jin G (2017) Unconfined compressive strength prediction from petrophysical properties and elemental spectroscopy using support-vector regression. In: Day 4 Thu, April 27, 2017. SPE. https://doi.org/10.2118/188077-MS
- Nooruddin HA, Anifowose F, Abdulraheem A (2014) Using soft computing techniques to predict corrected air permeability using Thomeer parameters, air porosity and grain density. Comput Geosci 64:72–80. https://doi.org/10.1016/j.cageo.2013.11.007ArticleGoogle Scholar
- Numbere OG, Azuibuike II, Ikiensikimama SS (2013) bubble point pressure prediction model for niger delta crude using artificial neural Network approach. In: Paper presented at the SPE Nigeria Annual international conference and exhibition, Lagos, Nigeria. https://doi.org/10.2118/167586-MS
- Oguntade T, Ojo T, Efajemue E, Oni B, Idaka J (2020) Application of ANN in predicting water based mud rheology and filtration properties. https://doi.org/10.2118/203720-MS
- Omar S, Ngadi A, Jebur HH (2013) machine learning techniques for anomaly detection: an overview. Int J Comput Appl 79:33–41. https://doi.org/10.5120/13715-1478ArticleGoogle Scholar
- Osarogiagbon A, Muojeke S, Venkatesan R, Khan F, Gillard P (2020) A new methodology for kick detection during petroleum drilling using long short-term memory recurrent neural network. Process Saf Environ Prot 142:126–137. https://doi.org/10.1016/j.psep.2020.05.046ArticleGoogle Scholar
- Osman EA, Aggour MA (2003) Determination of drilling mud density change with pressure and temperature made simple and accurate by ANN. In: Proceedings of the middle east oil show. https://doi.org/10.2118/81422-ms
- Osman EA, Abdel-Wahhab OA, Al-Marhoun MA (2001) Prediction of Oil PVT Properties Using Neural Networks. Paper presented at the SPE Middle East Oil Show, Manama, Bahrain. https://doi.org/10.2118/68233-MS
- Pandey SK, Janghel RR (2019) Recent deep learning techniques, challenges and its applications for medical healthcare system: a review. Neural Process Lett 50:1907–1935. https://doi.org/10.1007/s11063-018-09976-2ArticleGoogle Scholar
- Qi L, Carr TR (2006) Neural network prediction of carbonate lithofacies from well logs, big bow and sand arroyo creek fields, Southwest Kansas. Comput Geosci 32:947–964. https://doi.org/10.1016/j.cageo.2005.10.020ArticleGoogle Scholar
- Qi J, Li F, Marfurt K (2017) Multiazimuth coherence. Geophysics. https://doi.org/10.1190/GEO2017-0196.1ArticleGoogle Scholar
- Rabbani E, Sharif F, Koolivand Salooki M, Moradzadeh A (2012) Application of neural network technique for prediction of uniaxial compressive strength using reservoir formation properties. Int J Rock Mech Min Sci 56:100–111. https://doi.org/10.1016/j.ijrmms.2012.07.033ArticleGoogle Scholar
- Rahmanifard H, Plaksina T (2018) Application of fast analytical approach and AI optimization techniques to hydraulic fracture stage placement in shale gas reservoirs. J Nat Gas Sci Eng 52:367–378. https://doi.org/10.1016/j.jngse.2018.01.047ArticleGoogle Scholar
- Rahmati AS, Tatar A (2019) Application of radial basis function (RBF) neural networks to estimate oil field drilling fluid density at elevated pressures and temperatures. Oil Gas Sci Technol—Rev d’IFP Energies Nouv. https://doi.org/10.2516/ogst/2019021ArticleGoogle Scholar
- Raj J (2019) A comprehensive survey on the computational intelligence techniques and its applications. J ISMAC 1:147–159. https://doi.org/10.36548/jismac.2019.3.002ArticleGoogle Scholar
- Ramamoorthy A, Yampolskiy R (2018) Beyond map?: the race for artificial general Intelligence. ITU J 1(1):77–84 Google Scholar
- Ramirez AM, Valle GA, Romero F, Jaimes M (2017) Prediction of PVT properties in crude oil using machine learning techniques MLT. In: SPE Latin American and Caribbean petroleum engineering conference proceedings. Society of petroleum engineers. https://doi.org/10.2118/185536-ms
- Rasheed A, San O, Kvamsdal T (2020) Digital twin: values, challenges and enablers from a modeling perspective. IEEE Access 8:21980–22012. https://doi.org/10.1109/ACCESS.2020.2970143ArticleGoogle Scholar
- Rasheed Khan M, Tariq Z, Abdulraheem A (2018) Machine learning derived correlation to determine water saturation in complex lithologies. In: Society of petroleum engineers—SPE Kingdom of Saudi Arabia annual technical symposium and exhibition 2018, SATS 2018. Society of petroleum engineers. https://doi.org/10.2118/192307-ms
- Rastogi A, Sharma A (2019) Quantifying the impact of fracturing chemicals on production performance using machine learning. In: Paper presented at the SPE Liquids-Rich Basins conference—North America, Odessa, Texas, USA. https://doi.org/10.2118/197095-MS
- Razi MM, Mazidi M, Razi FM, Aligolzadeh H, Niazi S (2013) Artificial neural network modeling of plastic viscosity, yield point, and apparent viscosity for water-based drilling fluids. J Dispers Sci Technol. https://doi.org/10.1080/01932691.2012.704746ArticleGoogle Scholar
- Reiber F, Vos BE, Eide SE (1999) On-line torque & drag: a real-time drilling performance optimization tool. In: Proceedings of the IADC/SPE Asia Pacific drilling technology conference, APDT. https://doi.org/10.2523/52836-ms
- Rolon L, Mohaghegh SD, Ameri S, Gaskari R, McDaniel B (2009) Using artificial neural networks to generate synthetic well logs. J Nat Gas Sci Eng. https://doi.org/10.1016/j.jngse.2009.08.003ArticleGoogle Scholar
- Rooki R, Doulati Ardejani F, Moradzadeh A (2014) Hole cleaning prediction in foam drilling using artificial neural network and multiple linear regression. Geomaterials. https://doi.org/10.4236/gm.2014.41005ArticleGoogle Scholar
- Rooki R, Doulati Ardejani F, Moradzadeh A, Kelessidis VC, Nourozi M (2012) Prediction of terminal velocity of solid spheres falling through newtonian and non-newtonian pseudoplastic power law fluid using artificial neural network. Int J Miner Process. https://doi.org/10.1016/j.minpro.2012.03.012ArticleGoogle Scholar
- Safiuddin M, Raman SN, Salam MA, Jumaat MZ (2016) Modeling of compressive strength for self-consolidating high-strength concrete incorporating palm oil fuel ash. Materials (basel). https://doi.org/10.3390/ma9050396ArticleGoogle Scholar
- Sagheer A, Kotb M (2019) Time series forecasting of petroleum production using deep LSTM recurrent networks. Neurocomputing 323:203–213. https://doi.org/10.1016/j.neucom.2018.09.082ArticleGoogle Scholar
- Salehi SM, Honarvar B (2014) Automatic identification of formation iithology from well log data: a machine learning approach. J Pet Sci Res. https://doi.org/10.14355/jpsr.2014.0302.04ArticleGoogle Scholar
- Samek W, Wiegand T, Muller KR (2018) Explainable artificial intelligence: Understanding, visualizing and interpreting deep learning models. ITU J 1(1):39 Google Scholar
- Samnejad M, Gharib Shirangi M, Ettehadi R (2020) A digital twin of drilling fluids rheology for real-time rig operations . https://doi.org/10.4043/30738-MS
- Sebtosheikh MA, Salehi A (2015) Lithology prediction by support vector classifiers using inverted seismic attributes data and petrophysical logs as a new approach and investigation of training data set size effect on its performance in a heterogeneous carbonate reservoir. J Pet Sci Eng 134:143–149. https://doi.org/10.1016/j.petrol.2015.08.001ArticleGoogle Scholar
- Seyyedattar M, Ghiasi MM, Zendehboudi S, Butt S (2020) Determination of bubble point pressure and oil formation volume factor: extra trees compared with LSSVM-CSA hybrid and ANFIS models. Fuel 269:116834. https://doi.org/10.1016/j.fuel.2019.116834ArticleGoogle Scholar
- Shabbir J, Anwer T (2018) Artificial intelligence and its role in near future. J Latex Class Files 1(8):1–11 Google Scholar
- Shadravan A, Tarrahi M, Amani M (2017) Intelligent tool to design drilling, spacer, cement slurry, and fracturing fluids by use of machine-learning algorithms. SPE Drill Complet 32:131–140. https://doi.org/10.2118/175238-PAArticleGoogle Scholar
- Shahkarami A, Mohaghegh S, Gholami V, Haghighat A, Moreno D (2014) Modeling pressure and saturation distribution in a CO 2 storage project using a surrogate reservoir model (SRM). Greenh Gases Sci Technol 4:289–315. https://doi.org/10.1002/ghg.1414ArticleGoogle Scholar
- Shahriar A, Nehdi ML (2011) Modeling rheological properties of oil well cement slurries using artificial neural networks. J Mater Civ Eng. https://doi.org/10.1061/(ASCE)MT.1943-5533.0000340ArticleGoogle Scholar
- Sharma MSR, O’Regan M, Baxter CDP, Moran K, Vaziri H, Narayanasamy R (2010) Empirical relationship between strength and geophysical properties for weakly cemented formations. J Pet Sci Eng 72:134–142. https://doi.org/10.1016/j.petrol.2010.03.011ArticleGoogle Scholar
- Shojaei M-J, Bahrami E, Barati P, Riahi S (2014) Adaptive neuro-fuzzy approach for reservoir oil bubble point pressure estimation. J Nat Gas Sci Eng 20:214–220. https://doi.org/10.1016/j.jngse.2014.06.012ArticleGoogle Scholar
- Shokir EMEM (2004) Prediction of the hydrocarbon saturation in low resistivity formation via artificial neural network. In: Proceedings of the SPE Asia Pacific conference on integrated modelling for asset management. Society of petroleum engineers, pp. 35–40. https://doi.org/10.2118/87001-ms
- Shokrollahi A, Tatar A, Safari H (2015) On accurate determination of PVT properties in crude oil systems: committee machine intelligent system modeling approach. J Taiwan Inst Chem Eng 55:17–26. https://doi.org/10.1016/j.jtice.2015.04.009ArticleGoogle Scholar
- Van Si L, Chon BH (2018) Effective prediction and management of a CO2 flooding process for enhancing oil recovery using artificial neural networks. J Energy Resour Technol Trans ASME. https://doi.org/10.1115/1.4038054ArticleGoogle Scholar
- Sibai FN, Hosani HI, Naqbi RM, Dhanhani S, Shehhi S (2011) Iris recognition using artificial neural networks. Expert Syst Appl 38:5940–5946. https://doi.org/10.1016/j.eswa.2010.11.029ArticleGoogle Scholar
- Silva AA, Lima Neto IA, Misságia RM, Ceia MA, Carrasquilla AG, Archilha NL (2015) Artificial neural networks to support petrographic classification of carbonate-siliciclastic rocks using well logs and textural information. J Appl Geophys 117:118–125. https://doi.org/10.1016/j.jappgeo.2015.03.027ArticleGoogle Scholar
- Simandoux P (1963) Mesures dielectriques en milieu porexe, application a mesure des saturation eau. Inst Fr du Pet Suppl Issue
- Solomon O, Adewale D, Anyanwu C (2017) Fracture width prediction and loss prevention material sizing in depleted formations using artificial intelligence. In: Paper presented at the SPE Nigeria annual international conference and exhibition, Lagos, Nigeria. https://doi.org/10.2118/189068-MS
- Somasundaram S, Mund B, Soni R, Sharda R (2017) Seismic attribute analysis for fracture detection and porosity prediction: a case study from tight volcanic reservoirs, Barmer Basin, India. Lead Edge. https://doi.org/10.1190/tle36110947b1.1ArticleGoogle Scholar
- Somvanshi M, Chavan P, Tambade S, Shinde SV (2016) A review of machine learning techniques using decision tree and support vector machine. In: 2016 International conference on computing communication control and automation (ICCUBEA). IEEE, pp. 1–7. https://doi.org/10.1109/ICCUBEA.2016.7860040
- Srinivasan G, Hyman JD, Osthus DA, Moore BA, O’Malley D, Karra S, Rougier E, Hagberg AA, Hunter A, Viswanathan HS (2018) Quantifying topological uncertainty in fractured systems using graph theory and machine learning. Sci Rep. https://doi.org/10.1038/s41598-018-30117-1ArticleGoogle Scholar
- Sun H, Belhaj H, Tao G, Vega S, Liu L (2019) Rock properties evaluation for carbonate reservoir characterization with multi-scale digital rock images. J Pet Sci Eng 175:654–664. https://doi.org/10.1016/j.petrol.2018.12.075ArticleGoogle Scholar
- Sun Q, Ertekin T (2020) Screening and optimization of polymer flooding projects using artificial-neural-network (ANN) based proxies. J Pet Sci Eng. https://doi.org/10.1016/j.petrol.2019.106617ArticleGoogle Scholar
- Tahmasebi P, Javadpour F, Sahimi M (2017) Data mining and machine learning for identifying sweet spots in shale reservoirs. Expert Syst Appl 88:435–447. https://doi.org/10.1016/j.eswa.2017.07.015ArticleGoogle Scholar
- Tariq Z, Elkatatny S, Mahmoud M, Abdulraheem A (2016) A New artificial intelligence based empirical correlation to predict sonic travel time, In: International petroleum technology conference. International petroleum technology conference. https://doi.org/10.2523/iptc-19005-ms
- Tariq Z, Elkatatny S, Mahmoud M, Ali AZ, Abdulraheem A, (2017a) A new technique to develop rock strength correlation using artificial intelligence tools. In: Society of petroleum engineers—SPE reservoir characterisation and simulation conference and exhibition, RCSC 2017. Society of petroleum engineers, pp. 1340–1353. https://doi.org/10.2118/186062-MS
- Tariq Z, Elkatatny S, Mahmoud M, Ali AZ, Abdulraheem A (2017b) A new approach to predict failure parameters of carbonate rocks using artificial intelligence tools. In: Society of petroleum engineers—SPE Kingdom of Saudi Arabia annual technical symposium and exhibition 2017. Society of petroleum engineers, pp. 1428–1440. https://doi.org/10.2118/187974-MS
- Tariq Z (2018) An automated flowing bottom-hole pressure prediction for a vertical well having multiphase flow using computational intelligence techniques. In: Society of petroleum engineers—SPE Kingdom of Saudi Arabia annual technical symposium and exhibition 2018, SATS 2018. Society of petroleum engineers. https://doi.org/10.2118/192184-MS
- Tariq Z, Abdulraheem A, Mahmoud M, Ahmed A (2018) A rigorous data-driven approach to predict Poisson’s ratio of carbonate rocks using a functional network. Petrophysics 59:761–777. https://doi.org/10.30632/PJV59N6-2018a2ArticleGoogle Scholar
- Tariq Z, Mahmoud M, Abdulraheem A (2019) Core log integration: a hybrid intelligent data-driven solution to improve elastic parameter prediction. Neural Comput Appl. https://doi.org/10.1007/s00521-019-04101-3ArticleGoogle Scholar
- Tariq Z, Mahmoud M, Abdulraheem A (2020a) An intelligent data-driven model for dean-stark water saturation prediction in carbonate rocks. Neural Comput Appl 32:11919–11935. https://doi.org/10.1007/s00521-019-04674-zArticleGoogle Scholar
- Tariq Z, Murtaza M, Mahmoud M (2020b) Effects of nanoclay and silica flour on the mechanical properties of class G cement. ACS Omega 5:11643–11654. https://doi.org/10.1021/acsomega.0c00943ArticleGoogle Scholar
- Tariq Z, Murtaza M, Mahmoud M (2020c) Development of new rheological models for class G cement with nanoclay as an additive using machine learning techniques. ACS Omega 5:17646–17657. https://doi.org/10.1021/acsomega.0c02122ArticleGoogle Scholar
- Tariq Z, Mahmoud M, Abdulraheem A (2021) Machine learning-based improved pressure–volume–temperature correlations for black oil reservoirs. J Energy Resour Technol. https://doi.org/10.1115/1.4050579ArticleGoogle Scholar
- Taunk K, De S, Verma S, Swetapadma A (2019) A Brief review of nearest neighbor algorithm for learning and classification. In: 2019 International conference on intelligent computing and control systems (ICCS). IEEE, pp. 1255–1260. https://doi.org/10.1109/ICCS45141.2019.9065747
- Tian X, Daigle H (2019) Preferential mineral-microfracture association in intact and deformed shales detected by machine learning object detection. J Nat Gas Sci Eng 63:27–37. https://doi.org/10.1016/j.jngse.2019.01.003ArticleGoogle Scholar
- Tomiwa O, Oluwatosin R, Temiloluwa O, Oluwasanmi O, Joy I (2019) Improved water based mud using solanum tuberosum formulated biopolymer and application of artificial neural network in predicting mud rheological properties . https://doi.org/10.2118/198861-MS
- Trtnik G, Kavčič F, Turk G (2009) Prediction of concrete strength using ultrasonic pulse velocity and artificial neural networks. Ultrasonics. https://doi.org/10.1016/j.ultras.2008.05.001ArticleGoogle Scholar
- Tschannen V, Delescluse M, Ettrich N, Keuper J (2020) Extracting horizon surfaces from 3D seismic data using deep learning. Geophysics 85:N17–N26. https://doi.org/10.1190/geo2019-0569.1ArticleGoogle Scholar
- Van SL, Chon BH (2017b) Applicability of an artificial neural network for predictingwater-alternating-CO2 performance. Energies. https://doi.org/10.3390/en10070842ArticleGoogle Scholar
- Le Van S, Chon BH (2017a) Evaluating the critical performances of a CO2–Enhanced oil recovery process using artificial neural network models. J Pet Sci Eng. https://doi.org/10.1016/j.petrol.2017.07.034ArticleGoogle Scholar
- Virginia D (2018) Responsible artificial intelligence: designing AI for human values. ITU J 1(1):1–8 Google Scholar
- Wang S, Chen Z, Chen S (2019) Applicability of deep neural networks on production forecasting in Bakken shale reservoirs. J Pet Sci Eng 179:112–125. https://doi.org/10.1016/j.petrol.2019.04.016ArticleGoogle Scholar
- Wang Y, Duan M, Wang D, Liu J, Dong Y (2011) A model for deepwater floating platforms selection based on BP artificial neural networks
- Wattenbarger R, El-Banbi A, Villegas M, Maggard J (1998) Production analysis of linear flow into fractured tight gas wells. In: Paper SPE 39931 presented at the SPE rocky mountain regional/lowpermeability reservoirs symposium, Denver, Colorado, pp. 5–8 April. Paper Presented at the Low-permeability Reservoirs Symposium, Denver, CO
- Weyrauch T, Herstatt C (2016) What is frugal innovation? Three defining criteria. J Frugal Innov 2(1):1–17 ArticleGoogle Scholar
- Xiong W, Ji X, Ma Y, Wang Y, Benhassan NM, Ali MN, Luo Y (2018) Seismic fault detection with convolutional neural network. Geophysics. https://doi.org/10.1190/geo2017-0666.1ArticleGoogle Scholar
- YIlmaz I, Yuksek AG (2008) An example of artificial neural network (ANN) application for indirect estimation of rock parameters. Rock Mech Rock Eng 41:781–795. https://doi.org/10.1007/s00603-007-0138-7ArticleGoogle Scholar
- Yadollahi MM, Benli A, Demirboğa R (2015) Prediction of compressive strength of geopolymer composites using an artificial neural network. Mater Res Innov 19(6):453–458. https://doi.org/10.1179/1433075X15Y.0000000020ArticleGoogle Scholar
- Yang D, Rahman MK, Chen Y (2008) Bottomhole assembly analysis by finite difference differential method. Int J Numer Methods Eng. https://doi.org/10.1002/nme.2221ArticleGoogle Scholar
- You L, Tan Q, Kang Y, Xu C, Lin C (2018) Reconstruction and prediction of capillary pressure curve based on particle swarm optimization-back propagation neural network method. Petroleum 4:268–280. https://doi.org/10.1016/j.petlm.2018.03.004ArticleGoogle Scholar
- Yu W, Sepehrnoori K (2013) Optimization of multiple hydraulically fractured horizontal wells in unconventional gas reservoirs. In: SPE production and operations symposium, proceedings. https://doi.org/10.2118/164509-ms
- Zapico JL, Gonzlez-Buelga A, Gonzlez MP, Alonso R (2008) Finite element model updating of a small steel frame using neural networks. Smart Mater Struct. https://doi.org/10.1088/0964-1726/17/4/045016ArticleGoogle Scholar
- Zhao T, Jayaram V, Roy A, Marfurt KJ (2015) A comparison of classification techniques for seismic facies recognition. Interpretation. https://doi.org/10.1190/INT-2015-0044.1ArticleGoogle Scholar
- Zheng Y, Zhang Q, Yusifov A, Shi Y (2019) Applications of supervised deep learning for seismic interpretation and inversion. Lead Edge 38:526–533. https://doi.org/10.1190/tle38070526.1ArticleGoogle Scholar
- Zhou H, Niu X. Fan H, Wang G (2016) Effective calculation model of drilling fluids density and ESD for HTHP well while drilling. In: Society of petroleum engineers—IADC/SPE Asia Pacific drilling technology conference. https://doi.org/10.2118/180573-MS
- Zou G, Ren K, Sun Z, Peng S, Tang Y (2019) Fault interpretation using a support vector machine: A study based on 3D seismic mapping of the Zhaozhuang coal mine in the Qinshui Basin, China. J Appl Geophys. https://doi.org/10.1016/j.jappgeo.2019.103870ArticleGoogle Scholar
Author information
Authors and Affiliations
- King Fahd University of Petroleum and Minerals, Dhahran, Saudi Arabia Zeeshan Tariq, Murtada Saleh Aljawad, Amjed Hasan, Mobeen Murtaza, Emad Mohammed, Ammar El-Husseiny, Sulaiman A. Alarifi, Mohamed Mahmoud & Abdulazeez Abdulraheem
- Zeeshan Tariq